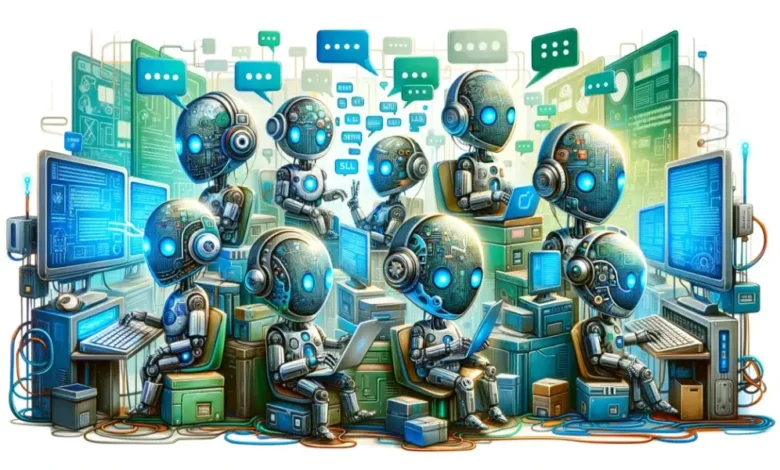
The race in which these big tech companies are offering to revamp artificial intelligence (AI) is driven by the massive capital that they are committing to maintaining and accelerating their large language models. Microsoft itself pledges $100 billion for its supercomputer programs, which are targeted for 2028 to be launched and a boost to its AI innovations.
These days, there is a relatively larger size in the model, and not to be forgotten, the small language models (SLMs) are also gaining popularity. These models test the familiar assumption of the NTG that the bigger, the better in the field of NOLP. SLMs, having word counts starting from a few million and going up to a few billion, are becoming paramount, especially in areas depicted by limited resources where they have been used successfully to do special tasks.
SLMs contribute to the performance parity with their bigger and more expensive rivals through improved training practices, procedures, and optimization methodology. Their ability range allows them to understand emotions, create gist, answer questions, and develop code. On-premise devices, mobile apps, and sites with low computational ability can also be the place where they are deployed.
One of the most famous circuits that Google and Microsoft use under their brand names, respectively, is Gemini Nano and Orca-2. The latest Google Pixel phones are supported by the former, whereas the latter is featured in the technologies manufactured by the latter. There are advantages of SLMs over large-scale models, as SLMs can host on-premises deployment for securing the data, faster inference speed/rate, less latency, and cost-effective comparatively.
New techniques are being discovered and applied every day, keeping the research and development process in mind, and in this way, SLMs turn out to be promising future trends. The foreseeable techniques, like the ones, will make this capability an advantage for them so they won’t lag behind the large models. SLMs are an amazing option for companies with high speed and efficacy with small money bags, specifically in skilled-oriented operations.